Nonlinearity Makes Photonic Neural Networks Smarter
Artificial intelligence (AI) based on neural networks aims to solve complex problems by emulating the human brain – and, much like the brain itself, it is full of surprises. It turns out, for example, that a very elegant way to create a neural network is to use random matrices. In other words, the links between the input and output of the network are assigned fixed and completely random weights. At the output, linear regression methods can then be used to train the network.
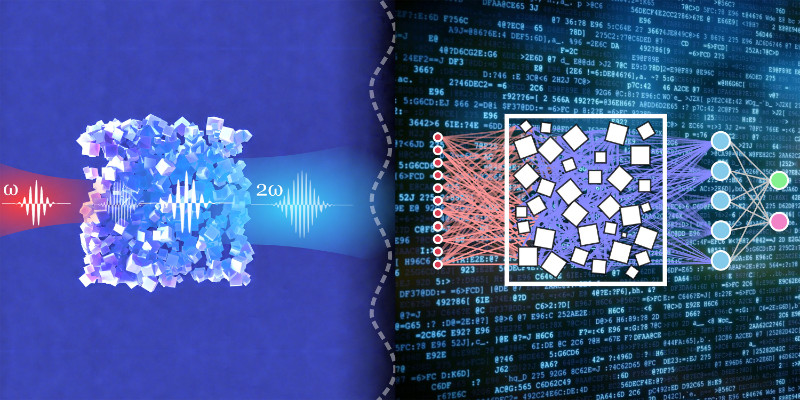
Whilst this is a powerful method on paper, implementing it on a computer can be tricky because of the memory requirements for large random matrices. Also, power consumption and computing time are likely to become an issue with digital computers as neural networks become larger and more complex. In recent years, researchers have tried to overcome these problems by using the natural physical equivalent of a random matrix – a disordered optical material – and realize the necessary matrix multiplication through light scattering.
Such photonic neuromorphic computers promise to overcome the weaknesses of digital computers mentioned above, but they also have a major drawback: photon scattering is a linear process. This means that the activation functions of the network nodes, which determine how the inputs to a node result in an output, are also linear. This, in turn, implies that the resulting neural networks are essentially single-layer networks and therefore, in AI jargon, not particularly “expressive”.
In a joint effort, a team of researchers led by Rachel Grange at the Institute for Quantum Electronics in Zurich and by Sylvain Gigan at the Laboratoire Kastler Brossel (LKB) in Paris, together with colleagues from Italy and China, have now shown that photonic neural networks can be made smarter by using a disordered material made of lots of tiny crystals that can double the frequency of incoming light in a nonlinear process. In their paper, recently published in Nature Computational Science, they demonstrate that their approach leads to a sizeable increase in performance over simple linear scattering.
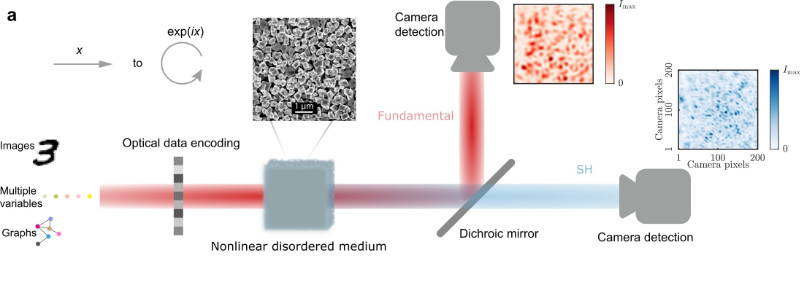
Core processing unit made of disordered nanoparticles
The task of the ETH researchers was to produce and characterize the core processing unit of the photonic neural network, which was then integrated into an experimental setup at the LKB. “Our group provided the samples and our expertise on optical nonlinearity”, says Alfonso Nardi, a postdoc working with Rachel Grange. He and his colleagues used tiny crystals of lithium niobate (LiNbO3) that had been chemically synthesized in such a way that the crystals had sizes between 100 and 400 nanometres. They deposited the suspension containing the crystals on a substrate. After the solvent had evaporated, a solid slab of 5 micrometre thickness containing randomly oriented nanocrystals was created.
Grange and her collaborators chose the material and particle size such that light scattering was maximized, to the point that the mean free path of photons in the slab was below one optical wavelength. At the same time, the lithium niobate crystals – which have a non-centrosymmetric crystal lattice and, therefore, a non-vanishing Chi-2 nonlinearity – can double the frequency of incoming light through a nonlinear process of second harmonic generation. Since they are randomly oriented, there is always global second harmonic emission regardless of the phase-matching conditions.
To feed data into the photonic network, the researchers at LKB used a spatial light modulator that converts images or numerical values into optical phases. A pulsed laser at 800 nm sends photons through that modulator, which are then scattered multiple times inside the lithium niobate slab. Through second harmonic generation, photons at 400 nm are created and also scattered. For both wavelengths, the multiple and phase-coherent scattering events lead to characteristic speckle patterns, which are separated by a dichroic mirror and then recorded on CCD cameras.
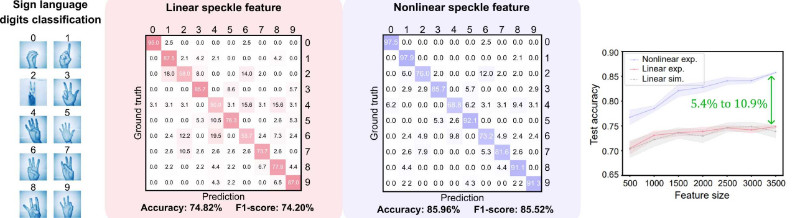
Superior performance thanks to nonlinearity
From the speckle patterns, the network was now trained using regression methods. To show the strength of their system, which effectively realized more than 27,000 input and 3,500 output nodes, the researchers applied it to a range of machine learning tasks from image recognition to graph classification. In one such task, the photonic neural network was trained to recognize sign language digits, in which the numbers from 0 to 9 are represented by different combinations of opened fingers. After the training, the researchers determined how many times the network got the right answer, using either the linearly scattered photons or those resulting from the nonlinear second harmonic generation. The result was clear: while the linear neural network accurately recognized the digits in around 74% of cases on average, the nonlinear network achieved a hit rate of close to 86%.
“This is a first important step towards establishing optical nonlinearity as a key factor for the future of photonic computing”, says postdoc Andrea Morandi. To further improve the energy efficiency of the photonic network, the researchers plan to use a continuous-wave rather than a pulsed laser. This could be achieved, for example, by using an optical cavity or by engineering novel nonlinear materials with a higher second-harmonic yield.